Visual AI Search - the latest game-changing solution
Lets focus on how the search function can be enhanced in DAMs using Visual AI Search, allowing users to search using natural language or visual cues, thereby bypassing the limitations of keywords and traditional metadata-based search methods.
This user-centric approach not only improves satisfaction and productivity but also ensures that the full value of the digital asset repository is realized.
Do DAM users today find what they are searching for using keywords and metadata?
Content in DAMs should be organised in a way that makes most sense. Ideally, it’s organised to be accessible, usable and efficient to find, and there’s an underlying structural logic, which will help users to navigate through information. Making everything findable becomes easier to navigate when a taxonomy leads our understanding.
A DAM system without a structured content organization makes locating specific digital assets a needle-in-a-haystack scenario. The key to avoiding such frustration and enhancing usability lies in well-organized content and metadata. But users today get sometimes frustrated by the search experience in DAMs. They feel distressed, lost and disoriented. The search experience is in many cases not good enough because the content is not well curated! Why is that?
If people say the DAM is hard to use, they’re probably right, as in some cases DAM admins have likely focused on making the system a great tool for themselves, but not for the end users of the DAM. In this case the DAM system was implemented without engaging users enough. On the other hand, content governance in a DAM system is a demanding task.
First it takes time to curate repositories, create keywords and taxonomies, either manually or automatically. DAM users invest valuable time in the content discovery process constructing intricate Boolean queries and filters to pinpoint the content they are looking for. And still, the user experience of searches hasn’t improved significantly. It always takes more time than it should. Frustrated by the complexity and poor search results, content providers and admins often simply give up before adding metadata which in the end makes searches a struggle for all users.
Generally speaking, you won’t get a good search result based on just keywords because people don’t think and describe the world in keywords. And, each person thinks differently. Different people use different ways to describe something or may write the same thing differently. People just do not think or search with keywords like girl, pasta, table, plate, glas, water, indoor, smile, at least it is no longer the only way! On the other hand teaching or expecting your users to understand a deep taxonomy or memorize a list of keywords used to describe visual assets is becoming impractical.
This is were Visual AI Search comes handy
The Visual AI Search feature is especially beneficial for quickly finding relevant images and videos. With the new Visual Search powered by generative AI, you can search for and find the perfect, hard to find image or video, without speculating on keywords or master taxonomy.
The new search understands natural language, context, abstract phrases, and even cultural references. It’s typo insensitive, so you can search faster. It can be configured to support many languages, allowing team members worldwide to find what they need quickly and easily.
Natural Language Queries: Users can search using conversational language, asking for what they need in the same way they would talk to a human. This eliminates the need to guess specific keywords or remember exact file names, making the search process more natural and intuitive.
Contextual Understanding: LLMs understand the context and nuances of user queries, allowing for more accurate and relevant search results. For example, a user looking for "bright and cheerful holiday-themed photos" will receive assets that precisely match the mood and theme, beyond just basic keyword matches.
Enabling AI Visual Search:
Users can enable and or choose AI Visual Search directly in the Search field, allowing for searches based on descriptive terms or phrases like "graduate students in the classroom" or "football keeper diving for a save." This flexibility makes it easier to find images that conceptually match the search query, regardless of the specific metadata tags assigned to them.
Using language models with free text enables a more accurate and attractive interface for users. AI Visual Search is simple to use. No more complex search terms. No learning curve. It has the familiar feel of a Google search and a prompt, but instead of being on the web, you’re searching for assets in your DAM.
Search Refinement and Exploration: Conversational language enable users to refine their searches using natural language follow-ups, making it easier to narrow down results or explore related concepts without starting from scratch. This iterative search process closely mimics human exploration and discovery, significantly enhancing user satisfaction.
Scenarious where Visual AI Search enhances searches
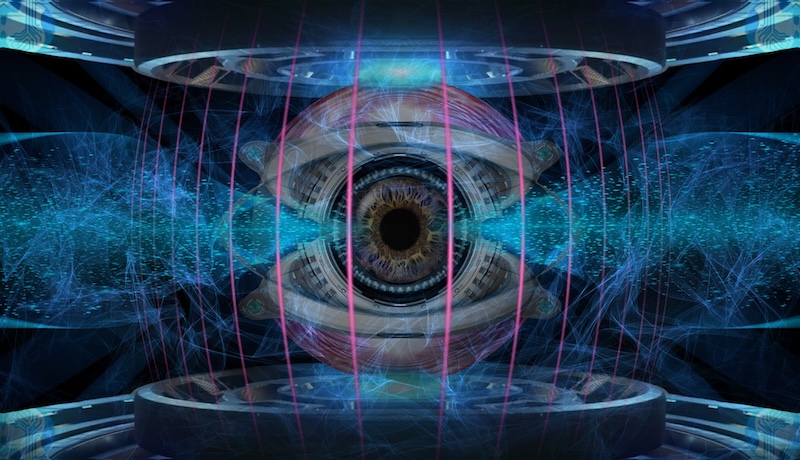
1.Great benefits for large asset repositories and/or new DAM-implementations
If the organisation needs a new DAM to be implemented fast and it includes a lot of imagery without well defined descriptions the usage of a Visual AI Search powered DAM might be the right choice. Instead of combing through poorly tagged files, AI can analyze the visual elements of assets, making it much easier to find what you need quickly. This means you can upload images and videos and find similar images in your database without any predefined tags or descriptions. This feature is especially beneficial when onboarding a new large repository, as all the assets are searchable immediately, and the speed of finding a visual drops to virtually zero.
Onboarding gets easy
Furthermore, in this scenario DAM users no longer need to rely on special training in searching or tagging procedures, so they can stop guessing and focus on their actual business goals. So, onboarding and integration are simple and fast. Ongoing management is simple, scalable, and highly cost-effective.
Visual Search without Metadata or incomplete Metadata: AI Visual Search technologies enable users to find relevant media using natural language or visual cues without relying on pre-existing metadata. This approach helps in discovering assets also with incomplete or inaccurate metadata and is particularly useful for managing large and diverse content libraries.
You can spend less time on tedious tagging and asset management work.
There’s no need to build a taxonomy (it’s embedded in any language model), add keywords, or train users. This feature significantly reduces the time and effort required for finding specific images, especially in large collections where assets may not be thoroughly tagged and can sometimes be incomplete or inaccurate.
Improved Asset Utilization
With AI visual search, previously underutilized assets are more likely to be discovered and used, as the search capabilities are not limited to text tags that may have been omitted or incorrectly applied.
This approach is great for AI powered new DAMs and general bigger repositories, but it also guides users not to adapt metadata workflows in their DAMs which might become a problem later on.
2. Expanding the traditional DAM functionality with Visual AI Search
Integrating Visual AI-powered search into Digital Asset Management (DAM) workflows can revolutionize how organizations store, retrieve, and manage their digital content. Here's how Visual AI Search integrates and operates within a traditional DAM workflow, along with how query results can be refined for later usage.
Combining Visual AI Search with Traditional DAM:
This allows users to start with a broad, natural language query using AI-powered Visual Search. Incorporate LLM-based searches as a starting point for searches before diving into specific collections curated by their teams. By complementing the search with keyword and metadata searches, enriches the data available for each asset. This hybrid approach ensures the efficiency of AI with the nuance and context understanding of human input.
AI Tagging and Metadata:
Although AI Visual Search operates independently of metadata, DAM providers may also support AI-based automatic tagging for images upon upload. This feature identifies specific people or brands in images, creating searchable metadata tags. This can complement the visual search by making it easier to locate images based on known entities or subjects, thus enhancing the asset management process further.
Search Similar:
Expand the Visual AI search result with a "Search Similar" function for an asset in the search results. Enabling it lets your users find other assets related to the initial asset based on context. With the a Similar Search button, anyone can explore the asset collection and get great results without becoming a keyword and metadata expert.
By identifying visually similar or duplicate content, Visual AI can help organizations reduce redundancy in their digital asset libraries. Visual AI toolscan flag duplicates or similar content for review. This streamlines storage and makes asset management more efficient.
Adding metadata descriptions manually:
Adding keywords to describe specific project-related elements or unique products makes sense. For example, metadata can include contextual information that AI might not recognize, such as the project for which an asset was used or specific campaign details. Project-related elements like “winter collection” or “created by Nora” are not part of the visual. Adding them to the description field is helpful to be able to search with those terms. The same goes for SKUs and other classification metadata.
There are ways to use both natural language, desciptions and keywords: The algorithms can live side by side or be combined.. This combination ensures a robust searchability framework that leverages both visual and contextual data. While the technology behind the integration is not trivial, the results are promising.
Visual AI Search - the latest game-changing solution
Visual AI Search plays a crucial role in larger Digital Asset Management (DAM) workflows by enhancing the efficiency and effectiveness of managing, retrieving, and utilizing digital assets such as images, videos, documents, and other media files. It means removing the barrier of keywords and taxonomy dependency in DAMs.
Enhanced by general AI-features
Other AI-powered solutions in the DAM may be utilized in parallell to Visual AI Search. With an AI-Platform you can avoid duplicates, find similar images, recognize persons, tag images, detect trademarks and work smarter. Find what you need, and save time and tears while doing so. Using language models enables a more accurate and attractive interface for your users.
With AI Visual Search, you can find the perfect images and videos in your DAM effortlessly using natural language — no metadata required. Free yourself and your teams from inaccurate metadata that can limit your search.
Modern media banks and DAMs are shifting towards AI-powered workflows
DAM providers approach to AI-driven search functionality demonstrates a shift towards more intelligent, efficient, and user-friendly digital asset management systems. By reducing dependency on manually entered metadata and making visual content more accessible through AI-driven search capabilities, DAM providers enable creative teams to save time, enhance productivity, and maximize the value of their digital assets.
Generative AI in visual content is revolutionizing the field as well as multiplying the creation of visual assets and thereby expanding content libraries. New AI models can create images, videos, and interactive media based on textual descriptions like text to image and recently text to video. In the process, businesses and workflows will be transformed dramatically and this applies also to digital asset management (DAM).
Training AI Models: To maximize the effectiveness of AI visual search, it is crucial to train AI models using existing assets and metadata. This training allows the AI to learn about the specific types of assets an organization uses and to refine its search capabilities based on real-world usage and feedback. This is where lots of local data is required and knowledge of the local data is eminent. As the workload might get too much to handle for smaller orgainisations help is availible by experienced vendors.
Best of both workflows
Integrating visual AI search with traditional DAM workflows that utilize metadata creates a dynamic, efficient, and highly effective asset management system that harnesses the best of both technological advancements and established practices. This synergy not only enhances the user experience but also maximizes the value of digital assets in supporting organizational goals and creative endeavors.
As a leading service provider we offer expertise in digital asset management (DAM) and management of sophisticated solutions to a variety of business areas. Communication Pro helps to build and customize DAM, that will get easy to use.
Interested? Check out our DAM services here or book a online meeting here.
![]() |
Author Rolf Koppatz Rolf is the CEO and consultant at Communication Pro with long experience in DAMs, Managing Visual Files, Marketing Portals, Content Hubs and Computer Vision. Contact me at LinkedIn. |